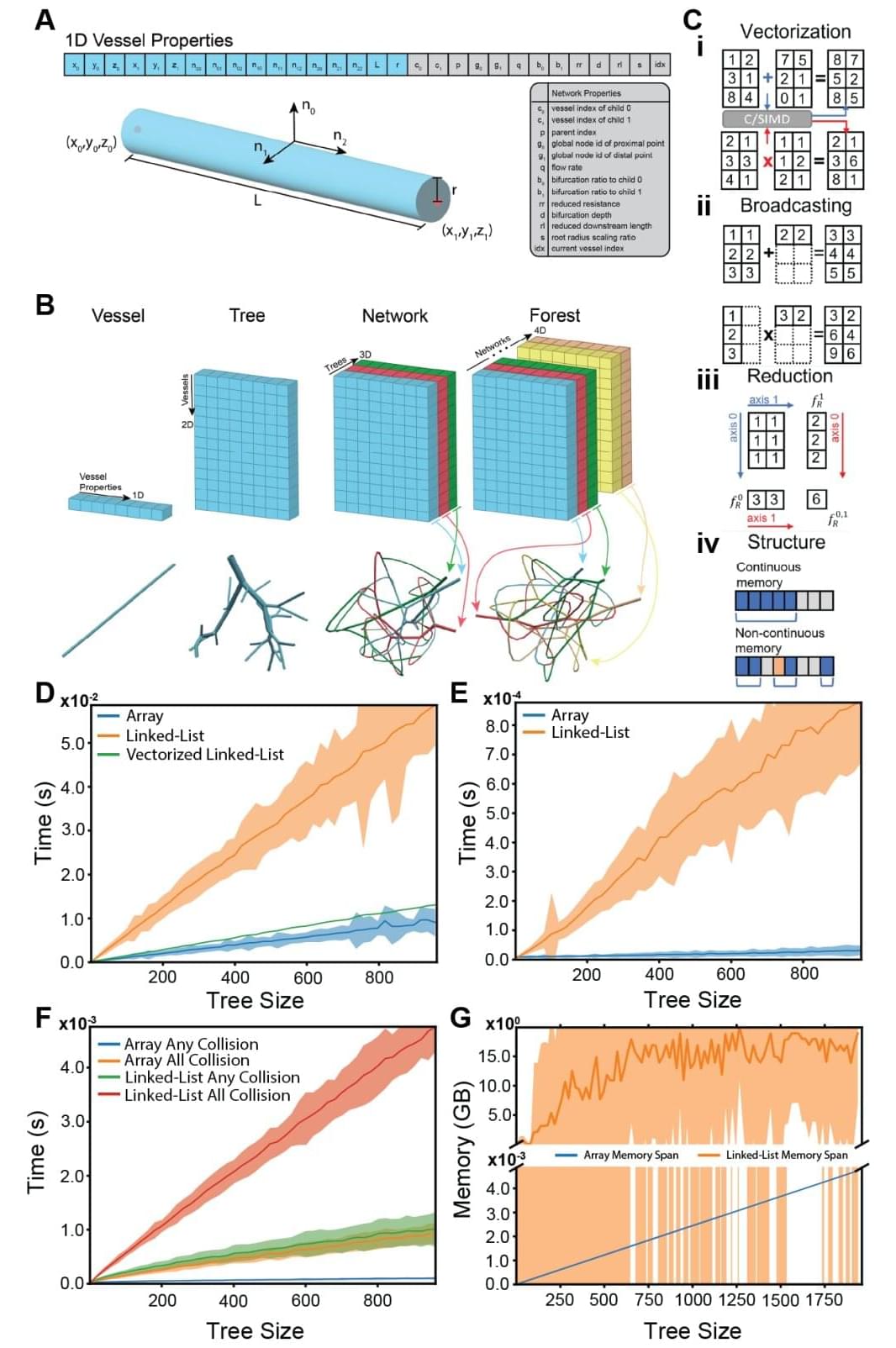
There are more than 100,000 people on organ transplant lists in the U.S., some of whom will wait years to receive one—and some may not survive the wait. Even with a good match, there is a chance that a person’s body will reject the organ. To shorten waiting periods and reduce the possibility of rejection, researchers in regenerative medicine are developing methods to use a patient’s own cells to fabricate personalized hearts, kidneys, livers, and other organs on demand.
Ensuring that oxygen and nutrients can reach every part of a newly grown organ is an ongoing challenge. Researchers at Stanford have created new tools to design and 3D print the incredibly complex vascular trees needed to carry blood throughout an organ. Their platform, published June 12 in Science, generates designs that resemble what we actually see in the human body significantly faster than previous attempts and is able to translate those designs into instructions for a 3D printer.
“The ability to scale up bioprinted tissues is currently limited by the ability to generate vasculature for them—you can’t scale up these tissues without providing a blood supply,” said Alison Marsden, the Douglas M. and Nola Leishman Professor of Cardiovascular Diseases, professor of pediatrics and of bioengineering at Stanford in the Schools of Engineering and Medicine and co-senior author on the paper. “We were able to make the algorithm for generating the vasculature run about 200 times faster than prior methods, and we can generate it for complex shapes, like organs.”