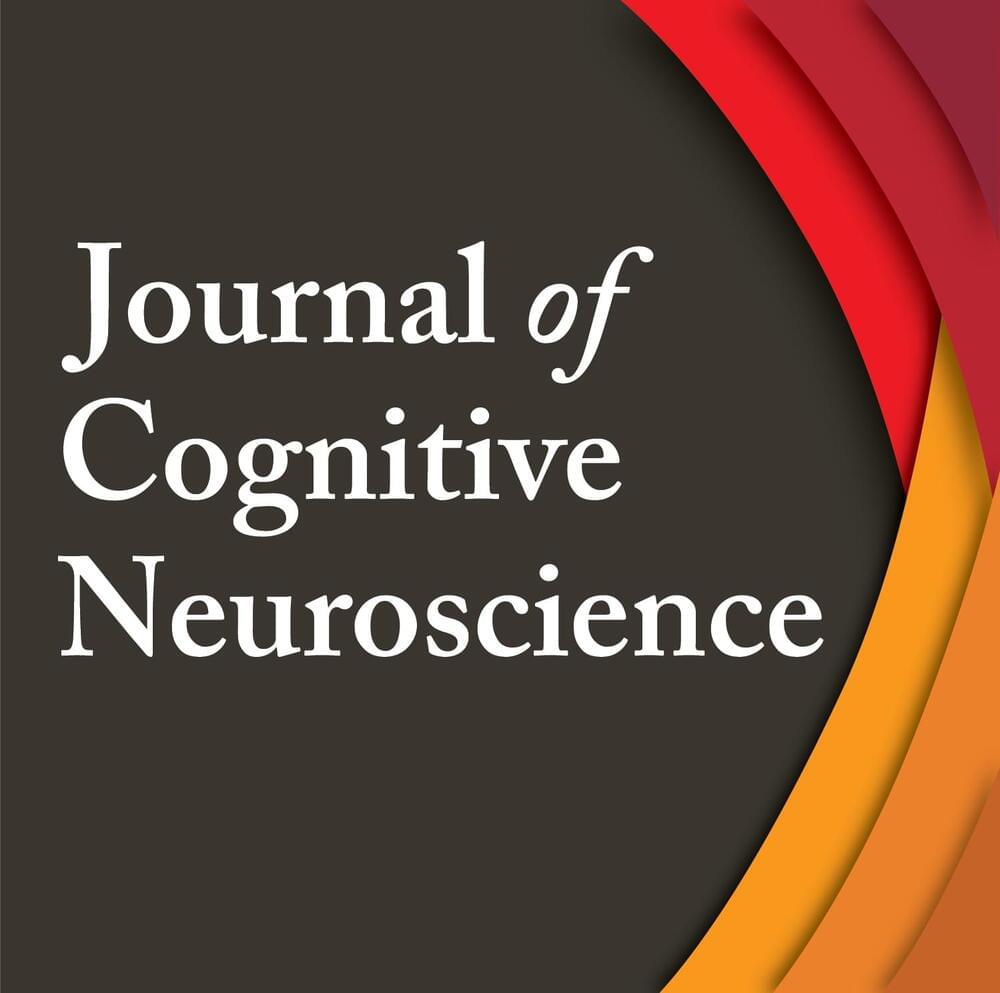
It is well known that the human brain is a complex system that comprises not only individual brain regions but also distributed neural networks. The human brain is efficiently organized by integrating information across various brain regions to minimize the cost of information processing while maximizing the overall efficiency of the brain networks. Modern neuroimaging techniques allow us to identify distinct local cortical regions and investigate large-scale neural networks underlying math competence both structurally and functionally. To gain insights into the neural bases of math competence, this review aims to find answers based on structural and functional properties of the human brain in both typical and atypical populations of children and adults. Specifically, for atypical populations, we will focus on individuals with math learning deficits. Math learning deficits are neurodevelopmental disorders that impair an individual’s ability to learn and perform math-related tasks. Dyscalculia is a specific type of math learning deficit that affects the development of arithmetical skills and other basic numerical skills (Kuhl, Sobotta, Legascreen Consortium, & Skeide, 2021; Kucian et al., 2014; Rykhlevskaia, Uddin, Kondos, & Menon, 2009). When reviewing findings from atypical population, we will focus on individuals with math learning deficits including those with dyscalculia.
As math competence encompasses many different skills, for studies involving adults, this review will selectively examine the neural bases of relatively complex math skills, such as evaluation of mathematical statements (e.g., “Any equilateral triangle can be divided into two right triangles”; Amalric & Dehaene, 2016, 2019). For studies involving children, we will also include fundamental math abilities such as arithmetic skills that are commensurate with the math skills young children master. However, basic number comprehension and number comparison skills are outside the scope of this review. Moreover, we will consider whether neural markers associated with math competence are unique to math or may be reflective of academic achievement and cognitive abilities more generally.