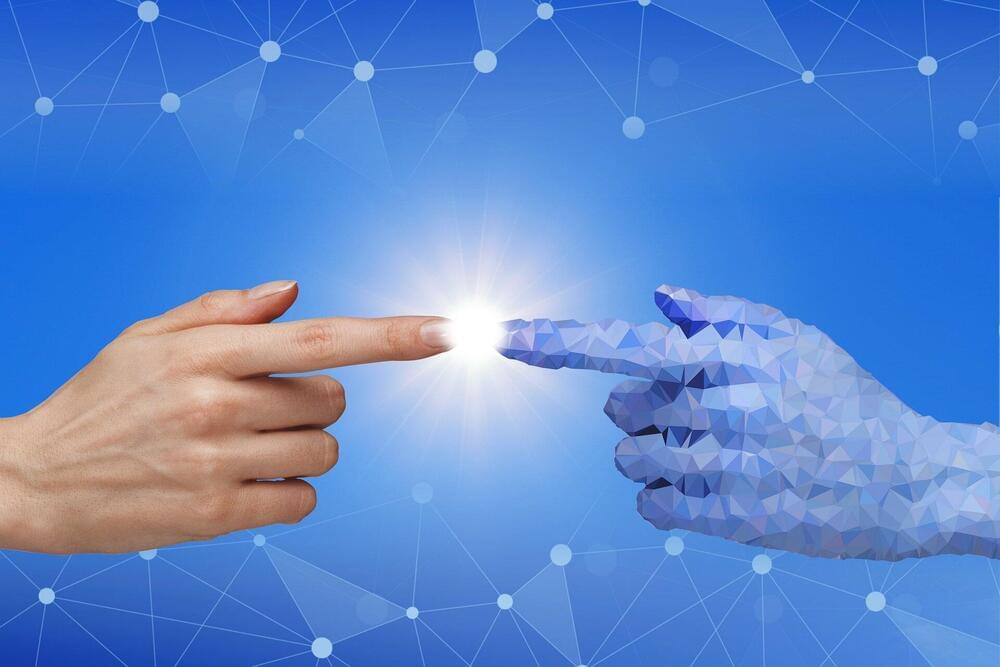
“We usually conform to the views of others for two reasons. First, we succumb to group pressure and want to gain social acceptance. Second, we lack sufficient knowledge and perceive the group as a source of a better interpretation of the current situation,” explains Dr. Konrad Bocian from the Institute of Psychology at SWPS University.
So far, only a few studies have investigated whether moral judgments, or evaluations of another person’s behavior in a given situation, are subject to group pressure. This issue was examined by scientists from SWPS University in collaboration with researchers from the University of Sussex and the University of Kent. The scientists also investigated how views about the behavior of others changed under the influence of avatar pressure in a virtual environment. A paper on this topic is published in PLOS ONE.
“Today, social influence is increasingly as potent in the digital world as in the real world. Therefore, it is necessary to determine how our judgments are shaped in the digital reality, where interactions take place online and some participants are avatars, not real humans,” points out Dr. Bocian.