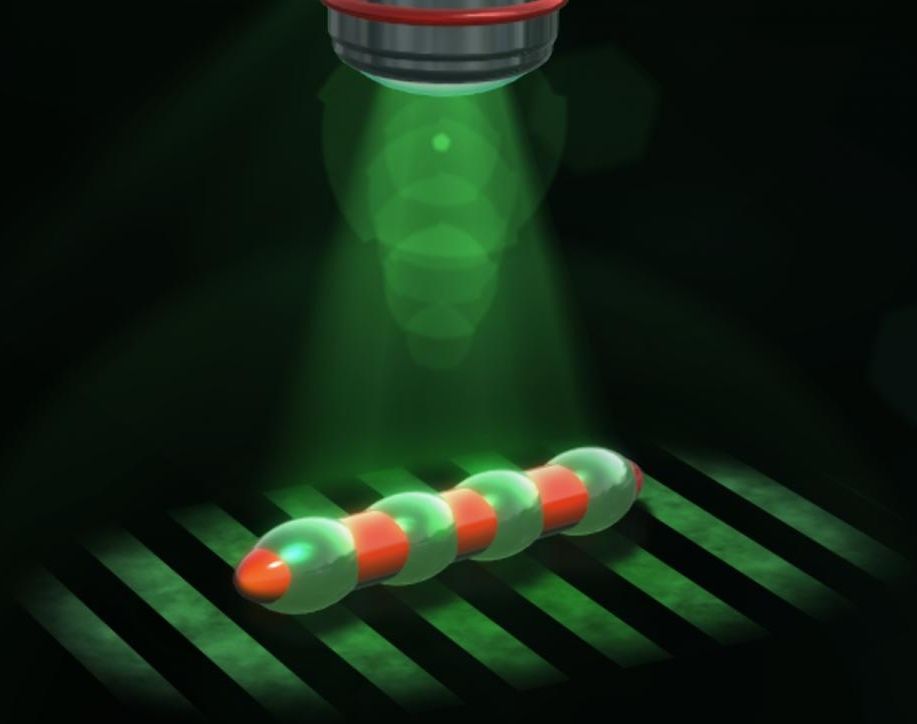
Ciliates can do amazing things: Being so tiny, the water in which they live is like thick honey to these microorganisms. In spite of this, however, they are able to self-propel through water by the synchronized movement of thousands of extremely thin filaments on their outer skin, called cilia. Researchers from the Max Planck Institute for Intelligent Systems in Stuttgart are now moving robots that are barely perceptible to the human eye in a similar manner through liquids. For these microswimmers, the scientists are neither employing complex driving elements nor external forces such as magnetic fields. The team of scientists headed by Peer Fischer have built a ciliate-inspired model using a material that combines the properties of liquid crystals and elastic rubbers, rendering the body capable of self-propelling upon exposure to green light. Mini submarines navigating the human body and detecting and curing diseases may still be the stuff of science fiction, but applications for the new development in Stuttgart could see the light-powered materials take the form of tiny medical assistants at the end of an endoscope.
Their tiny size makes life extremely difficult for swimming microorganisms. As their movement has virtually no momentum, the friction between the water and their outer skin slows them down considerably — much like trying to swim through thick honey. The viscosity of the medium also prevents the formation of turbulences, something that could transfer the force to the water and thereby drive the swimmer. For this reason, the filaments beat in a coordinated wave-like movement that runs along the entire body of the single-celled organism, similar to the legs of a centipede. These waves move the liquid along with them so that the ciliate — measuring roughly 100 micrometres, i.e. a tenth of a millimetre, as thick as a human hair — moves through the liquid.
“Our aim was to imitate this type of movement with a microrobot,” says Stefano Palagi, first author of the study at the Max Planck Institute for Intelligent Systems in Stuttgart, which also included collaborating scientists from the Universities of Cambridge, Stuttgart and Florence. Fischer, who is also a Professor for Physical Chemistry at the University of Stuttgart, states that it would be virtually impossible to build a mechanical machine at the length scale of the ciliate that also replicates its movement, as it would need to have hundreds of individual actuators, not to mention their control and energy supply.
Read more