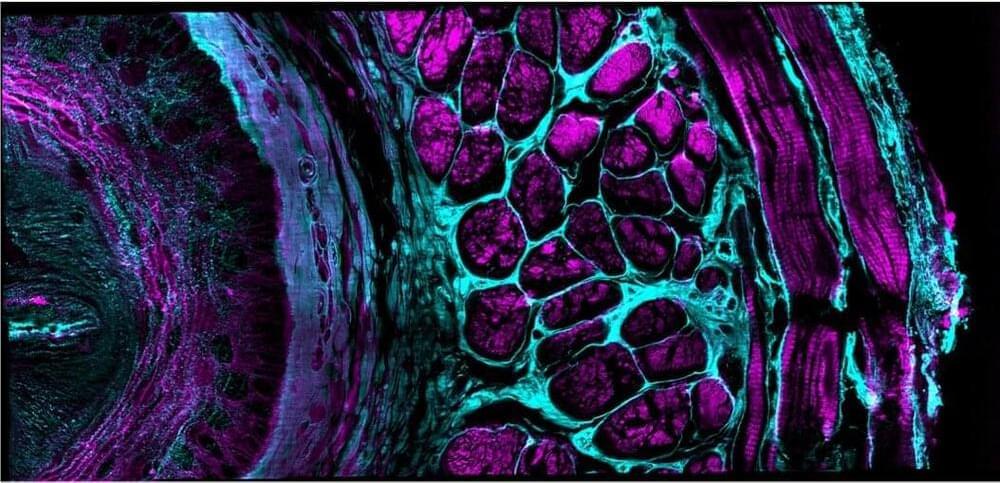
Since artificial intelligence pioneer Marvin Minsky patented the principle of confocal microscopy in 1957, it has become the workhorse standard in life science laboratories worldwide, due to its superior contrast over traditional wide-field microscopy. Yet confocal microscopes aren’t perfect. They boost resolution by imaging just one, single, in-focus point at a time, so it can take quite a while to scan an entire, delicate biological sample, exposing it light dosages that can be toxic.
To push confocal imaging to an unprecedented level of performance, a collaboration at the Marine Biological Laboratory (MBL) has invented a “kitchen sink” confocal platform that borrows solutions from other high-powered imaging systems, adds a unifying thread of “Deep Learning” artificial intelligence algorithms, and successfully improves the confocal’s volumetric resolution by more than 10-fold while simultaneously reducing phototoxicity. Their report on the technology, called “Multiview Confocal Super-Resolution Microscopy,” is published online this week in Nature.
“Many labs have confocals, and if they can eke more performance out of them using these artificial intelligence algorithms, then they don’t have to invest in a whole new microscope. To me, that’s one of the best and most exciting reasons to adopt these AI methods,” said senior author and MBL Fellow Hari Shroff of the National Institute of Biomedical Imaging and Bioengineering.