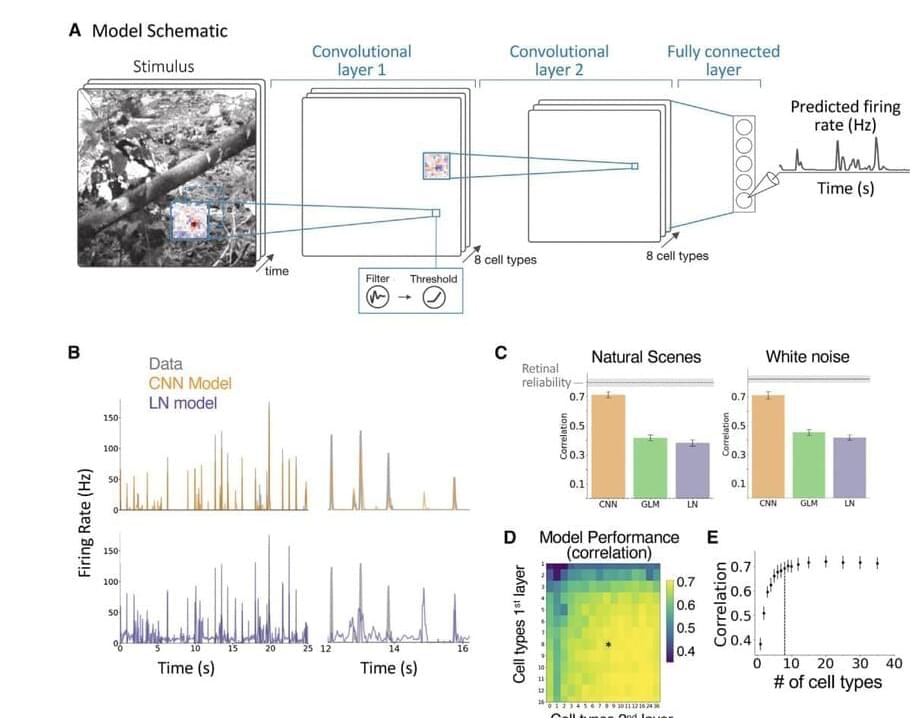
A fundamental goal in the field of sensory neuroscience is to understand the complex mechanisms that underlie the neural code responsible for processing natural visual scenes. In neuroscience, a fundamental yet unresolved question is how neural circuits are developed in natural settings by the interaction of multiple cell types. The eyes have evolved to communicate information about natural visual scenes using a wide range of interneurons, which is crucial for transmitting visual information to the brain.
Retina’s functioning is largely based on research into how it reacts to artificial stimuli like flashing lights and noise. These might not accurately represent how the retina interprets actual visual data. The complexity of how these more than 50 different types of interneurons contribute to retinal processing has yet to be fully understood despite the fact that different computations have been detected using such methods. In a recent research paper, a group of researchers has made a significant advancement by showing that a three-layer network model is capable of predicting retinal responses to natural sceneries with amazing precision, almost exceeding the bounds of experimental data. The researchers wanted to understand how the brain processes natural visual scenes, so they focussed on the retina, which is part of the eye that sends signals to the brain.
This model’s interpretability, i.e., the ability to comprehend and examine its internal organization, is one of its key characteristics. There is a strong correlation between the responses of interneurons that were directly included in the model and those that were separately recorded. This suggests that the model captures significant aspects of the retinal interneuron activity. It successfully reproduces a wide range of motion analysis, adaptability, and predictive coding phenomena when they are just trained on natural scenes. On the other hand, models trained on white noise cannot reproduce the same set of events, supporting the idea that examining natural sceneries is necessary to comprehend natural visual processing.